Cardiac event detection in echocardiography with triplane data and deep learning
This project demonstrates deep learning methods (3D CNN + LSTM) to detect six events in the cardiac cycle, thus adding more clinical information compared to previous timing networks using only two events. These additional events separate distinct physiological phases which may provide a less naïve representation of the cardiac cycle. We train this network on triplane recordings which provide multiple views of the heart with optimal synchronization for improved annotation accuracy.
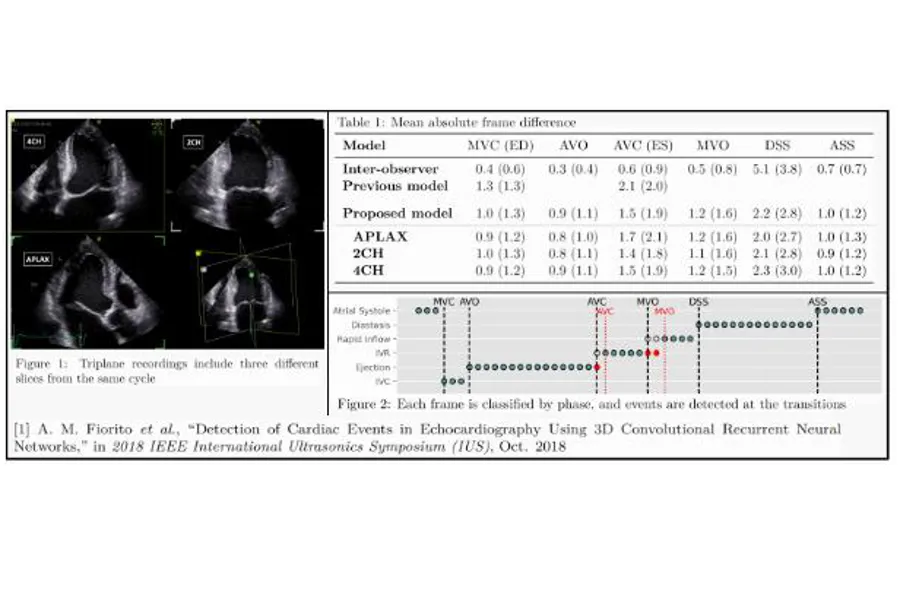
The work was presented as a poster at the IEEE International Ultrasonics Symposium (IUS) 2022. The project is still ongoing, and the goal is to extend to a full paper and test integration in 2023.
Related Researchers:
Fermann BS
Aase SA
Nyberg J
Grue JF
Grenne B,
Dalen H,
Remme E,
Løvstakken L,
Østvik A