Cardiomyopathy Prediction with Foundation Models
The research focuses on applying deep learning techniques to cardiac ultrasound imaging. We are investigating the capabilities of foundation models and self-supervised learning to automate measurements from echocardiograms, assess image quality, and improve diagnostic support. This work will help extend these models to new use cases in cardiac ultrasound, including the detection, characterization and progression study of cardiomyopathy.
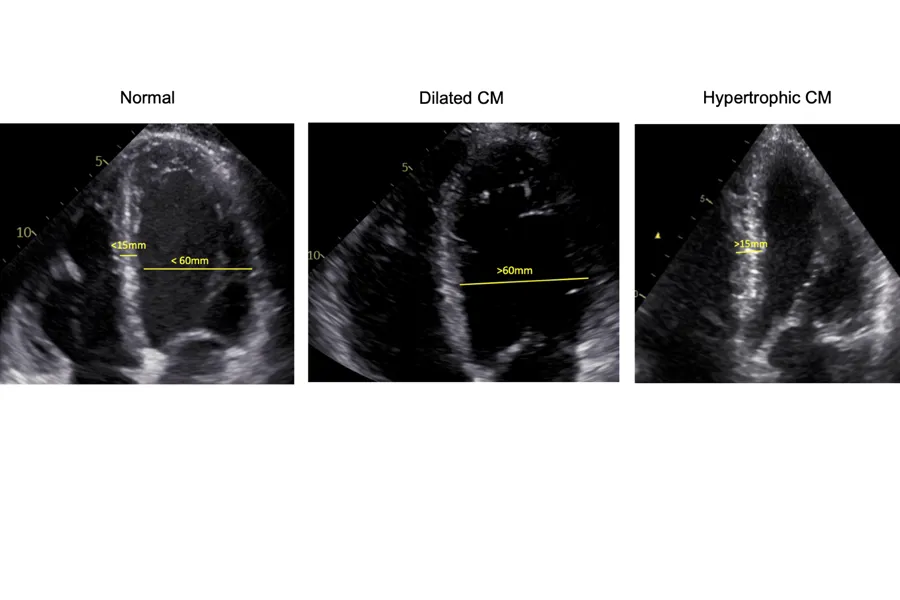
The objective is to develop and validate deep learning models for diagnosing cardiomyopathy using cardiac ultrasound imaging, with a focus on hypertrophic and dilated cardiomyopathy. By leveraging self-supervised learning and foundation models, we aim to enhance diagnostic accuracy, automate measurements, and explore image-based assessments for tracking disease progression.
Researchers involved
- Preetraj Bhoodoo, PhD fellow, UiO
- Main supervisor: Anna Solberg, Professor, UiO
- Co-supervisor: Sarina Thomas, Associate professor, UiO/GE HealthCare
- Erik Steen, Chief Engineer, GE HealthCare
- Nina Eide Hasselberg, MD/PhD, OUS
- Paul Olsen, MD/PhD fellow, OUS/UiO
- Christian K. Five, MD/PhD fellow, OUS
- Lasse Løvstakken, Professor, NTNU
- Elisabeth Wetzer, Associate professor, UiT
Collaboration